Method of image filtering using singular decomposition and the surrogate data technology
DOI:
https://doi.org/10.3103/S0735272716090041Keywords:
additive noise, filtering, singular decomposition, spatial resolution, surrogate dataAbstract
A method for nonlinear filtering of additive noise on digital image has been proposed. This method is based on presenting the image by its matrix singular decomposition and applying the surrogate data technology to components of the image. The proposed method ensures a superior resolution as compared to most common methods of window filtering that is corroborated by the results of simulation modeling.References
GONZALEZ, R.C.; WOODS, R.E. Digital Image Processing, 2nd ed. Prentice Hall, 2002.
FISENKO, V.T.; FISENKO, T.Y. Computer Image Processing and Image Recognition. St. Petersburg: SPbGU ITMO, 2008 [in Russian].
GRUZMAN, I.S.; KIRICHUK, V.S. Digital Processing of Images in Information Systems. Novosibirsk: NGTU, 2002 [in Russian].
VITKUS, R.Y.; YAROSLAVSKII, L.P. Adaptive Linear Filters for Image Processing. Adaptive Methods of Image Processing. Moscow: Nauka, 1988 [in Russian, ed. by V. I. Siforov, L. P. Yaroslavskii].
SOLODUSHKIN, V.I.; UDOD, V.A. One-dimensional image filtering, optimal with respect to resolution. Atmosph. Oceanic Optics, 1991, v.4, n.10, p.720-722, http://ao.iao.ru/en/content/vol.4-1991/iss.10/4.
RODIONOV, S.A. Estimation of optic image quality, in the Reference Book: Single-Dimensional Computational Optics. Leningrad: Mashinostroyeniye, 1984 [in Russian].
SHIRMAN, Y.D. Resolution and Compression of Signals. Moscow: Sov. Radio, 1974 [in Russian].
KOSTENKO, P.Y.; VASYLYSHYN, V.I.; SLOBODYANYUK, V.V. Additive noise reduction in digital images using the surrogate data technology. Syst. Obrob. Inf., 2014, n.8, p.33-38.
KANTZ, H.; SCHREIBER, T. Nonlinear Time Series Analysis. Cambridge: University Press, 2004.
SMALL, M. Applied Nonlinear Time Series Analysis Applications in Physics, Physiology and Finance. World Scientific Publishing Co. Pte. Ltd., 2005.
EFRON, B. Nonconventional Methods of Multivariate Statistical Analysis. Moscow: Finansy i Statistika, 1988 [in Russian, translation from English of articles collection], 263 p.
KOSTENKO, P.Y.; VASYUTA, K.S.; SLOBODYANYUK, V.V.; YAKOVENKO, D.S. The use of surrogate signals for enhancing the estimation quality of parameters of regular and chaotic signals observed against the background of additive noise. Systems of Control, Navigation and Communications, 2010, n.4, p.28-32.
KOSTENKO, P.Y.; VASYLYSHYN, V.I. Signal processing correction in spectral analysis using the surrogate autocovariance observation functions obtained by the ATS-algorithm. Radioelectron. Commun. Syst., 2014, v.57, n.6, p.235243, DOI: http://dx.doi.org/10.3103/S0735272714060016.
KOSTENKO, P.Y.; VASYLYSHYN, V.I. Enhancing the efficiency of spectral analysis of signals by the Root-MUSIC method using surrogate data. Radioelectron. Commun. Syst., 2014, v.57, n.1, p.31-38, DOI: http://dx.doi.org/10.3103/S0735272714010026.
SLOBODYANYUK, V.V.; SHAPOVALOV, O.V. Analysis of the impact of the scanning type of noisy digital image on the efficiency of noise suppression method using the surrogate data technology. Syst. Obrob. Inf., 2015, n.5, p.22-27.
DANILOV, D.L.; ZHIGLYAVSKII, A.A. Main Components of Time Series: Caterpillar Method. St. Petersburg: SPbGU, 1997 [in Russian].
GERSHMAN, A.B.; BOHME, JOHANN F. Improved DOA estimation via pseudorandom resampling of spatial spectrum. IEEE Signal Process. Lett., Feb. 1997, v.4, n.2, p.54-57, DOI: http://dx.doi.org/10.1109/97.554472.
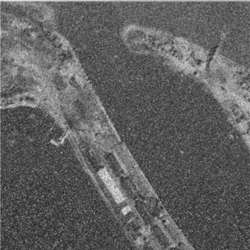