Intrahemispheric symmetry of brain perfusion. Part 1. Calculation procedure
DOI:
https://doi.org/10.3103/S073527272108001XKeywords:
single-photon emission computed tomography, SPECT, brain, rotation symmetry, intrahemispheric symmetry, perfusion, 99mTc-HMPAOAbstract
As has been noted in the analysis of the symmetry of radiopharmaceutical (RPh) distribution between the cerebral hemispheres according to the data of a single-photon emission computed tomography (SPECT), there are quasi-symmetric regions of perfusion in the hemispheres. This study is focused on the investigation of this observation that eventually will lead to the development of new quantitative criteria of the brain functional state in terms of effective perfusion, and possibly will provide new knowledge on the structural and functional patterns of the brain. The authors develop a methodology for estimating intrahemispheric symmetry (IHS) of brain perfusion based on SPECT data. 32 SPECT images of patients with different levels of brain perfusion were analyzed. Scintigraphic investigations of the brain were conducted with 99mTc-HMPAO on the gamma camera E.Cam (Siemens) with LEHR collimator. The SPECT investigation was conducted 15–20 minutes after the RPh injection. The tomography study included the collection of 128 projections for 128×128 matrix; the injected RPh activity amounted to 740 mBq. The developed computerized standardization procedure of spatial orientation of brain SPECT image was implemented using the Scintybrain software in the Matlab 2018 environment. The main criterion for finding quasi-symmetric lines of brain perfusion profile is the cross-correlation coefficient r and the standard deviation between them. The number of selected pairs of profile lines depends on the predetermined threshold rmin. The analysis of experimental data shows that it is recommended to adopt the rmin value equal to 0.94 for quantifying the brain IHS. This paper presents a hypothesis of intrahemispheric quasi-symmetry of brain perfusion. The developed method of IHS analysis provides a new diagnostic information about the spatial RPh distribution and represents a fundamentally new tool for assessing the interrelationships of circulatory disorders of brain tissues in its various segments.
References
D. C. Costa, “Single photon emission tomography (SPET) with 99Tc m-hexamethylpropyleneamineoxime (HMPAO) in research and clinical practice - a useful tool,” Vasc. Med. Rev., vol. vmr-1, no. 2, pp. 179–201, 1990, doi: https://doi.org/10.1177/1358836X9000100207.
J. W. Choi, M. H. Kim, S.-A. Park, D. S. Sin, M.-S. Kim, “Neural correlates of motor recovery measured by SPECT at six months after basal ganglia stroke,” Ann. Rehabil. Med., vol. 41, no. 6, p. 905, 2017, doi: https://doi.org/10.5535/arm.2017.41.6.905.
D. S. Liebeskind, “Imaging the future of stroke: I. Ischemia,” Ann. Neurol., vol. 66, no. 5, pp. 574–590, 2009, doi: https://doi.org/10.1002/ana.21787.
B. H. Brinkmann et al., “Statistical parametric mapping demonstrates asymmetric uptake with Tc-99m ECD and Tc-99m HMPAO SPECT in normal brain,” J. Cereb. Blood Flow Metab., vol. 32, no. 1, pp. 190–198, 2012, doi: https://doi.org/10.1038/jcbfm.2011.123.
K. M. Kang et al., “Correlation of asymmetry indices measured by arterial spin-labeling MR imaging and SPECT in patients with crossed cerebellar diaschisis,” Am. J. Neuroradiol., vol. 36, no. 9, pp. 1662–1668, 2015, doi: https://doi.org/10.3174/ajnr.A4366.
A. K. Kondakov, “Radionuklidnyie metodyi izucheniya perfuzii golovnogo mozga v diagnostike i kontrole kachestva lecheniya degenerativnyih i funktsionalnyih porazheniy nervnoy sistemy,” Moscow, 2018. uri: https://docplayer.ru/85071986-Kondakov-anton-kirillovich-luchevaya-diagnostika-luchevaya-terapiya.html.
A. W. Toga, P. M. Thompson, “Mapping brain asymmetry,” Nat. Rev. Neurosci., vol. 4, no. 1, pp. 37–48, 2003, doi: https://doi.org/10.1038/nrn1009.
M. LeMay, “Morphological cerebral asymmetries of modern man, fossil man, and nonhuman primate,” Ann. New York Acad. Sci., vol. 280, no. 1 Origins and E, pp. 349–366, 1976, doi: https://doi.org/10.1111/j.1749-6632.1976.tb25499.x.
J. M. Mountz, E. C. San Pedro, “Basis and clinical application of brain imaging,” in The Pathophysiologic Basis of Nuclear Medicine, Berlin: Springer Berlin Heidelberg, 2006, pp. 448–485.
T. Makazlieva et al., “Our preliminary experience with SPECT 99m Tc-HMPAO brain perfusion scans in diagnosis of dementia,” Acta Morphol., vol. 12, no. 1, pp. 22–28, 2015, uri: http://hdl.handle.net/20.500.12188/8102.
M. A. Viergever et al., “Registration, segmentation, and visualization of multimodal brain images,” Comput. Med. Imaging Graph., vol. 25, no. 2, pp. 147–151, 2001, doi: https://doi.org/10.1016/S0895-6111(00)00065-3.
C. Grova et al., “A methodology for generating normal and pathological brain perfusion SPECT images for evaluation of MRI/SPECT fusion methods: application in epilepsy,” Phys. Med. Biol., vol. 48, no. 24, pp. 4023–4043, 2003, doi: https://doi.org/10.1088/0031-9155/48/24/003.
L. Lazli, M. Boukadoum, O. A. Mohamed, “A survey on computer-aided diagnosis of brain disorders through MRI based on machine learning and data mining methodologies with an emphasis on Alzheimer disease diagnosis and the contribution of the multimodal fusion,” Appl. Sci., vol. 10, no. 5, p. 1894, 2020, doi: https://doi.org/10.3390/app10051894.
N. Nikolov, S. Makeev, T. Novikova, V. Tsikalo, “Spatial standardization of spect brain images with perfusion radiopharmaceuticals,” Innov. Biosyst. Bioeng., vol. 4, no. 2, pp. 67–74, 2020, doi: https://doi.org/10.20535/ibb.2020.4.2.195546.
A. Baldassarre, L. E. Ramsey, J. S. Siegel, G. L. Shulman, M. Corbetta, “Brain connectivity and neurological disorders after stroke,” Curr. Opin. Neurol., vol. 29, no. 6, pp. 706–713, 2016, doi: https://doi.org/10.1097/WCO.0000000000000396.
X. Guo et al., “Altered inter‐ and intrahemispheric functional connectivity dynamics in autistic children,” Hum. Brain Mapp., vol. 41, no. 2, pp. 419–428, 2020, doi: https://doi.org/10.1002/hbm.24812.
P. A. Robinson, “The balanced and introspective brain,” J. R. Soc. Interface, vol. 14, no. 130, p. 20160994, 2017, doi: https://doi.org/10.1098/rsif.2016.0994.
D. Kliemann et al., “Intrinsic functional connectivity of the brain in adults with a single cerebral hemisphere,” Cell Reports, vol. 29, no. 8, pp. 2398-2407.e4, 2019, doi: https://doi.org/10.1016/j.celrep.2019.10.067.
M. Filippi et al., “Brain network connectivity differs in early-onset neurodegenerative dementia,” Neurology, vol. 89, no. 17, pp. 1764–1772, 2017, doi: https://doi.org/10.1212/WNL.0000000000004577.
X. Ma et al., “Brain connectivity variation topography associated with working memory,” PLOS ONE, vol. 11, no. 12, p. e0165168, 2016, doi: https://doi.org/10.1371/journal.pone.0165168.
N. A. Nikolov et al., “Determination of absolute cerebral blood flow scintigraphy with lipophilic radiopharmaceutical,” Med. Phys., no. 3, pp. 36–45, 2018, uri: https://elibrary.ru/item.asp?id=35664229.
T. Carmen, “Do children with aggressive behavior have temporal lobe changes?,” Alasbimn J., vol. 5, no. 19, pp. AJ19-3, 2003, uri: http://web.uchile.cl/vignette/alasbimn2/alasbimn/CDA/sec_b/0,1206,SCID%253D2837,00.html.
M. Schmidt et al., “DSC brain perfusion using advanced deconvolution models in the diagnostic work-up of dementia and mild cognitive impairment: a semiquantitative comparison with HMPAO-SPECT-brain perfusion,” J. Clin. Med., vol. 9, no. 6, p. 1800, 2020, doi: https://doi.org/10.3390/jcm9061800.
X. Zheng, W. Wei, Q. Huang, S. Song, J. Wan, G. Huang, “A computer-aided analysis method of SPECT brain images for quantitative treatment monitoring: performance evaluations and clinical applications,” BioMed Res. Int., vol. 2017, pp. 1–11, 2017, doi: https://doi.org/10.1155/2017/1962181.
S. Cascianelli et al., “Role of artificial intelligence techniques (automatic classifiers) in molecular imaging modalities in neurodegenerative diseases,” Curr. Alzheimer Res., vol. 14, no. 2, pp. 198–207, 2017, doi: https://doi.org/10.2174/1567205013666160620122926.
Y. Höller et al., “Combining SPECT and quantitative EEG analysis for the automated differential diagnosis of disorders with amnestic symptoms,” Front. Aging Neurosci., vol. 9, 2017, doi: https://doi.org/10.3389/fnagi.2017.00290.
F. J. Martinez-Murcia et al., “Functional brain imaging synthesis based on image decomposition and kernel modeling: application to neurodegenerative diseases,” Front. Neuroinformatics, vol. 11, 2017, doi: https://doi.org/10.3389/fninf.2017.00065.
N. A. Nikolov, T. H. Novikova, S. S. Makeуev, “Assessment of informative value of effective cerebral blood flow calculation technique according to 99mTc-HMPAO polyphase scintigraphy data,” Ukr. J. Radiol. Oncol., vol. 29, no. 2, pp. 62–75, 2021, doi: https://doi.org/10.46879/ukroj.2.2021.62-75.
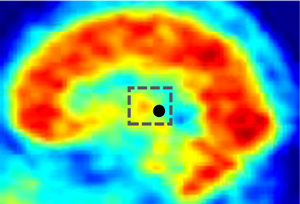