Presenting AR model error in terms of Geman-McClure function for prediction of processes in telecommunications
DOI:
https://doi.org/10.3103/S0735272722090023Keywords:
LPC, CELP, Geman-McClure function, autoregressive modelAbstract
Specific features of using the Geman-McClure function have been analyzed that are based on its properties in predicting telecommunication processes with anomalies or deviations by using an autoregressive model AR(p). The proposed modification of model AR(p) involves the presenting of prediction error in Geman-McClure metric that is based on this function and subsequent determining of coefficients of AR(p) model in this metric by employing equations presented in this paper that are similar to the Yule-Walker equations in presenting the prediction error of AR(p) model in L2 metric. Based on the comparative analysis and simulation, it has been established that AR(p) model in the Geman-McClure metric as compared with the classic AR(p) model in L2 metric makes it possible to enhance the prediction accuracy in telecommunication processes with anomalies or deviations by the factor of up to 1.5, while the efficiency of its use increases with the rise of model order p and the degree of process correlation that is subject to prediction. It has been shown that the practical use in telecommunications of the proposed modification of AR(p) model in the Geman-McClure metric is the most effective and expedient for the long-term prediction (large values of model order p) of strongly correlated processes that can be characterized by the presence of anomalies or deviations at relatively large values of internal parameter of this metric that ensures the speed of calculations in predicting the processes without a significant deterioration of its accuracy.
References
O. I. Pavlov, F. F. Dubrovka, “Evaluation of potential efficiency of speech coding using different parameters of linear prediction,” Radioelectron. Commun. Syst., vol. 63, no. 9, pp. 449–464, 2020, doi: https://doi.org/10.3103/S0735272720090010.
R. de Frein, “Power-weighted LPC formant estimation,” IEEE Trans. Circuits Syst. II Express Briefs, vol. 68, no. 6, pp. 2207–2211, 2021, doi: https://doi.org/10.1109/TCSII.2020.3040194.
C. O. Mawalim, S. Wang, M. Unoki, “Speech information hiding by modification of LSF quantization index in CELP codec,” in 2020 Asia-Pacific Signal and Information Processing Association Annual Summit and Conference (APSIPA ASC), 2020, uri: https://ieeexplore.ieee.org/document/9306401.
F. A. Semire, A. J. Adekunle, R. O. Abolade, O. A. Adegbola, “Prediction of rain attenuation trend due to climate change in some locations of Southwestern Nigeria,” Radioelectron. Commun. Syst., vol. 64, no. 1, pp. 45–52, 2021, doi: https://doi.org/10.3103/S0735272721010052.
P. J. Brockwell, R. A. Davis, Time Series: Theory and Methods. New York, NY: Springer New York, 1991, doi: https://doi.org/10.1007/978-1-4419-0320-4.
D. Ryabko, Asymptotic Nonparametric Statistical Analysis of Stationary Time Series. Cham: Springer International Publishing, 2019, doi: https://doi.org/10.1007/978-3-030-12564-6.
G. Kitagawa, Introduction to Time Series Modeling. New York: Chapman and Hall/CRC, 2020, doi: https://doi.org/10.1201/9780429197963.
V. V. Savchenko, “Method for reduction of speech signal autoregression model for speech transmission systems on low-speed communication channels,” Radioelectron. Commun. Syst., vol. 64, no. 11, pp. 592–603, 2021, doi: https://doi.org/10.3103/S0735272721110030.
Y. Zhang, N. Meratnia, P. Havinga, “Outlier detection techniques for wireless sensor networks: a survey,” IEEE Commun. Surv. Tutorials, vol. 12, no. 2, pp. 159–170, 2010, doi: https://doi.org/10.1109/SURV.2010.021510.00088.
D. M. Hawkins, Identification of Outliers. Dordrecht: Springer Netherlands, 1980, doi: https://doi.org/10.1007/978-94-015-3994-4.
V. Barnett, L. Toby, Outliers in Statistical Data. New York: Wiley, 1994, uri: https://www.wiley.com/en-us/Outliers+in+Statistical+Data%2C+3rd+Edition-p-9780471930945.
L. Wang, J. Yang, M. Workman, P. Wan, “Effective algorithms to detect stepping-stone intrusion by removing outliers of packet RTTs,” Tsinghua Sci. Technol., vol. 27, no. 2, pp. 432–442, 2022, doi: https://doi.org/10.26599/TST.2021.9010041.
L. Zou, Z. Wang, J. Hu, H. Dong, “Ultimately bounded filtering subject to impulsive measurement outliers,” IEEE Trans. Autom. Control, vol. 67, no. 1, pp. 304–319, 2022, doi: https://doi.org/10.1109/TAC.2021.3081256.
M. Kumar, M. Yadav, A. Chauhan, “Outlier analysis based intrusion detection for IoT,” in 2021 3rd International Conference on Advances in Computing, Communication Control and Networking (ICAC3N), 2021, pp. 1341–1348, doi: https://doi.org/10.1109/ICAC3N53548.2021.9725490.
N. Das, R. Bhattacharya, “Optimal sensor precision for multirate sensing for bounded estimation error,” IEEE Trans. Aerosp. Electron. Syst., vol. 58, no. 2, pp. 844–854, 2022, doi: https://doi.org/10.1109/TAES.2021.3111726.
A. V. Savchenko, V. V. Savchenko, “Scale-invariant modification of COSH distance for measuring speech signal distortions in real-time mode,” Radioelectron. Commun. Syst., vol. 64, no. 6, pp. 300–309, 2021, doi: https://doi.org/10.3103/S0735272721060030.
Y.-R. Feng, X.-C. Wei, L. Ding, T.-H. Song, R. X.-K. Gao, “A hybrid Schatten p -norm and Lp -norm with plane wave expansion method for near–near field transformation,” IEEE Trans. Electromagn. Compat., vol. 63, no. 6, pp. 2074–2081, 2021, doi: https://doi.org/10.1109/TEMC.2021.3083665.
S. Geman, D. McClure, “Statistical methods for tomographic image reconstruction,” in Proceeding of 46th Session of the ICI, Bulletin of the International Statistical Institute, 1987, vol. 52, no. 4, pp. 5–21.
H. Carfantan, J. Idier, “Statistical linear destriping of satellite-based pushbroom-type images,” IEEE Trans. Geosci. Remote Sens., vol. 48, no. 4, pp. 1860–1871, 2010, doi: https://doi.org/10.1109/TGRS.2009.2033587.
J. Chen, Z. Cai, X. Xie, J. Lai, “Motion estimation with L0 norm regularization,” in 2021 IEEE 7th International Conference on Virtual Reality (ICVR), 2021, pp. 127–134, doi: https://doi.org/10.1109/ICVR51878.2021.9483834.
E. A. Elsayed, Reliability Engineering. NJ: Wiley, 2020, doi: https://doi.org/10.1002/9781119665946.
G. L. Steele, S. Vigna, “Computationally easy, spectrally good multipliers for congruential pseudorandom number generators,” Softw. Pract. Exp., vol. 52, no. 2, pp. 443–458, 2022, doi: https://doi.org/10.1002/spe.3030.
J. C. Willems, “Recursive filtering,” Stat. Neerl., vol. 32, no. 1, pp. 1–39, 1978, doi: https://doi.org/10.1111/j.1467-9574.1978.tb01382.x.
W.-C. Tu, S.-Y. Chien, “Two-way recursive filtering,” IEEE Trans. Circuits Syst. Video Technol., vol. 31, no. 11, pp. 4255–4268, 2021, doi: https://doi.org/10.1109/TCSVT.2021.3049833.
A. G. Holubnychyi, G. F. Konakhovych, R. S. Odarchenko, “Signal constructions with low resultant sidelobes for pulse compression navigation and radar systems,” in 2016 4th International Conference on Methods and Systems of Navigation and Motion Control (MSNMC), 2016, pp. 267–270, doi: https://doi.org/10.1109/MSNMC.2016.7783158.
A. G. Holubnychyi, G. F. Konakhovych, A. G. Taranenko, Y. I. Gabrousenko, “Comparison of additive and multiplicative complementary sequences for navigation and flight control systems,” in 2018 IEEE 5th International Conference on Methods and Systems of Navigation and Motion Control (MSNMC), 2018, pp. 24–27, doi: https://doi.org/10.1109/MSNMC.2018.8576275.
A. H. Holubnychyi, G. F. Konakhovych, “Multiplicative complementary binary signal-code constructions,” Radioelectron. Commun. Syst., vol. 61, no. 10, pp. 431–443, 2018, doi: https://doi.org/10.3103/S0735272718100011.
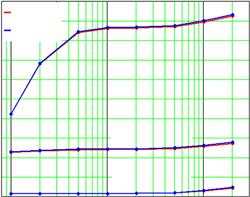