Wavelet filtering of signals without using model functions
DOI:
https://doi.org/10.3103/S0735272722020042Keywords:
recursive algorithm, root-mean-square error, numerical optimization, model signal, discrete wavelet transformation, noise filtering, decomposition levelAbstract
The effective wavelet filtering of real signals is impossible without determining their shape. The shape of a real signal is related to its wavelet spectrum. For shape analysis, a continuous color wavelet spectrogram of signal level is often used. The disadvantage of continuous wavelet spectrogram is the complexity of analyzing a blurry color image. A real signal with additive noise strongly distorts the spectrogram based on continuous wavelet analysis compared to a pure signal. Therefore, the identification of a real signal by using a continuous color wavelet spectrogram is difficult. To solve this problem, for the first time, a comparative analysis of spectrograms of signals and correlation matrices is carried out. The spectrograms of signals are obtained based on continuous wavelet transformation in the form of images with areas of different colors of variable intensity. Correlation matrices are computed by using mathematical functions of the coefficients of discrete wavelet spectra.
References
Y. N. Klikushin, V. Y. Kobenko, “Fundamentals of identification measurements,” Radio Electron. J., no. 5, 2006, uri: http://jre.cplire.ru/iso/nov06/index.html.
A. K. Lagirvandze, A. N. Kalinichenko, T. V. Morgunova, “ECG cycles forms analysis based on machine learning techniques,” Model. Syst. Networks Econ. Technol. Nature, Soc., no. 4, pp. 75–84, 2019, uri: https://cyberleninka.ru/article/n/algoritm-analiza-form-kardiotsiklov-ekg-s-ispolzovaniem-tehnologiy-mashinnogo-obucheniya/viewer.
D. A. Kuzin, L. G. Statsenko, P. N. Anisimov, M. M. Smirnova, “Applying machine learning methods to acoustic signal classification using spectrum characteristics,” Izv. SPBGETU “LETI,” no. 3, pp. 48–53, 2021.
M. S. Salman, A. Eleyan, B. Al-Sheikh, “Discrete-wavelet-transform recursive inverse algorithm using second-order estimation of the autocorrelation matrix,” TELKOMNIKA (Telecommunication Comput. Electron. Control., vol. 18, no. 6, p. 3073, 2020, doi: https://doi.org/10.12928/telkomnika.v18i6.16191.
T. Hu, J. Zhao, S. Yan, W. Zhang, “Performance analysis of a wavelet packet transform applied to concrete ultrasonic detection signals,” J. Phys. Conf. Ser., vol. 1894, no. 112062, 2021, doi: https://doi.org/10.1088/1742-6596/1894/1/012062.
V. O. Braun, V. P. Dolgushin, V. N. Loza, I. V. Pampukha, “Investigation of possibilities and characteristics of methods for reducing noise level in signal processing based on use of wavelet technology,” Radio Electron. J., no. 7, 2014, uri: http://jre.cplire.ru/jre/jul14/6/text.html.
Y. K. Taranenko, V. V. Lopatin, O. Y. Oliynyk, “Wavelet filtering by using nonthreshold method and example of model Doppler function,” Radioelectron. Commun. Syst., vol. 64, no. 7, pp. 380–389, 2021, doi: https://doi.org/10.3103/S0735272721070049.
G. Galati, G. Pavan, F. De Palo, “Chirp signals and noisy waveforms for solid-state surveillance radars,” Aerospace, vol. 4, no. 1, p. 15, 2017, doi: https://doi.org/10.3390/aerospace4010015.
D. O. Hogenboom, C. A. DiMarzio, “Quadrature detection of a Doppler signal,” Appl. Opt., vol. 37, no. 13, p. 2569, 1998, doi: https://doi.org/10.1364/AO.37.002569.
L. Debnath, “The Gabor Transform and Time-Frequency Signal Analysis,” in Wavelet Transforms and Their Applications, Boston, MA: Birkhäuser Boston, 2002, pp. 257–306.
M. Kovačević, “Signaling to relativistic observers: an Einstein–Shannon–Riemann encounter,” Probl. Inf. Transm., vol. 56, no. 4, pp. 303–308, 2020, doi: https://doi.org/10.1134/S0032946020040018.
P. Virtanen et al., “SciPy 1.0: fundamental algorithms for scientific computing in Python,” Nat. Methods, vol. 17, no. 3, pp. 261–272, 2020, doi: https://doi.org/10.1038/s41592-019-0686-2.
S. K. Goh, H. A. Abbass, K. C. Tan, A. Al-Mamun, C. Wang, C. Guan, “Automatic EEG artifact removal techniques by detecting influential independent components,” IEEE Trans. Emerg. Top. Comput. Intell., vol. 1, no. 4, pp. 270–279, 2017, doi: https://doi.org/10.1109/TETCI.2017.2690913.
B. Belkacemi, S. Saad, Z. Ghemari, F. Zaamouche, A. Khazzane, “Detection of induction motor improper bearing lubrication by discrete wavelet transforms (DWT) decomposition,” Instrum. Mes. Métrologie, vol. 19, no. 5, pp. 347–354, 2020, doi: https://doi.org/10.18280/i2m.190504.
Y. K. Taranenko, “Methods of discrete wavelet filtering of measuring signals: an algorithm for choosing a method,” Izmer. Tekhnika, no. 10, pp. 14–20, 2021, doi: https://doi.org/10.32446/0368-1025it.2021-10-14-20.
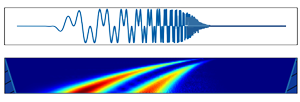