Method of image denoising in generalized phase space with improved indicator of spatial resolution
DOI:
https://doi.org/10.3103/S0735272719070045Keywords:
additive noise, phase space, surrogate data technologyAbstract
The paper proposes a nonlocal method of additive noise suppression in digital image based on presenting the image in matrix phase space and using nonconventional methods of multivariate statistical analysis, namely, the surrogate data technology that makes it possible to generate a pseudo-ensemble of surrogate images with their subsequent averaging using a single snapshot. This approach is based on properties of the coherent accumulation of signal component of the observation and noncoherent accumulation of its noise component as the size of observation ensemble increases that allows us to partially solve the contradiction between the denoising level and the distortion or loss of small-sized details of image, i.e. reduction of spatial resolution. The simulation modeling of the proposed method of generalized SDT-filtering of noise was conducted using the application software package of MathCad and Matlab. A comparative analysis of the spatial resolution of the proposed and several known methods of denoising has been carried out using the resolution–measurement criterion and the modified Rayleigh criterion. As is shown, the proposed method demonstrates a better spatial resolution as compared to the most common methods of denoising that is confirmed by the results of simulation modeling.References
BUADES, A.; COLL, B.; MOREL, J.-M. “A non-local algorithm for image denoising,” Proc. of 2005 IEEE Computer Society Conf. on Computer Vision and Pattern Recognition, CVPR’05, 20-25 June 2005, San Diego, USA. IEEE, 2005, v.2, p.60-65. DOI: https://doi.org/10.1109/CVPR.2005.38.
SOTO, M.A.; RAMIREZ, J.A.; THEVENAZ, L. “Optimizing image denoising for long-range Brillouin distributed fiber sensing,” J. Lightwave Technology, v.36, n.4, p.1168, Feb. 2018. DOI: https://doi.org/10.1109/JLT.2017.2750398.
GONZALEZ, R.C.; WOODS, R.E. Digital Image Processing, 2nd ed. Prentice Hall, 2002.
GRUZMAN, I.S.; KIRICHUK, V.S. Digital Processing of Images in Information Systems [in Russian]. Novosibirsk: NGTU, 2002.
EFRON, B. Nonconventional Methods of Multivariate Statistical Analysis [in Russian, translation from English of articles collection]. Moscow: Finansy i Statistika, 1988.
KOSTENKO, P.Yu.; SLOBODYANUK, V.V.; PLAHOTENKO, O.V. “Method of image filtering using singular decomposition and the surrogate data technology,” Radioelectron. Commun. Syst., v.59, n.9, p.409, 2016. DOI: https://doi.org/10.3103/S0735272716090041.
KOSTENKO, P.Yu.; VASYLYSHYN, V.I. “Surrogate data generation technology using the SSA method for enhancing the effectiveness of signal spectral analysis,” Radioelectron. Commun. Syst., v.58, n.8, p.356, 2015. DOI: https://doi.org/10.3103/S0735272715080038.
PIRONDINI, E.; VYBORNOVA, A.; COSCIA, M.; VAN DE VILLE, D. “A spectral method for generating surrogate graph signals,” IEEE Signal Processing Lett., v.23, n.9, p.1275, Sept. 2016. DOI: https://doi.org/10.1109/LSP.2016.2594072.
RABINOVICH, M.I.; FABRIKANT, A.L.; TSIMRING, L.Sh. “Finite-dimensional spatial disorder,” Sov. Phys. Usp., v.35, n.8, p.629, 1992. DOI: https://doi.org/10.1070/PU1992v035n08ABEH002253.
GERSHMAN, A.B.; BOHME, J.F. “Improved DOA estimation via pseudorandom resampling of spatial spectrum,” IEEE Signal Process. Lett., v.4, n.2, p.54, Feb. 1997. DOI: https://doi.org/10.1109/97.554472.
SHIRMAN, Ya.D. Resolution and Compression of Signals [in Russian]. Moscow: Sov. Radio, 1974.
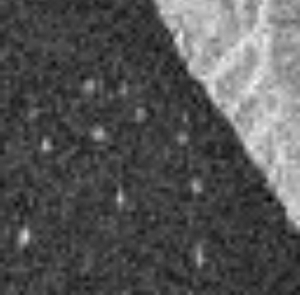