Clutter parameter estimation based on indirect algorithms
DOI:
https://doi.org/10.3103/S0735272719010060Keywords:
estimation algorithm, maximum likelihood method, sum-difference algorithm, correlation coefficient, Doppler phase shift, clutter, estimation accuracyAbstract
Indirect algorithms for clutter parameter estimation using linear transformations of initial data have been synthesized by the maximum likelihood method. The likelihood function was introduced for the input samples transformed in accordance with the sum-difference algorithms. The estimation algorithms of the interperiod correlation and Doppler phase shift coefficients derived by solving the appropriate likelihood equations do not contain the traditional complex multiplication operation over input data. The functional block diagram of the estimator of appropriate clutter parameters that can be used in adaptive rejection filters is presented. The estimation accuracy analysis of required parameters of clutter is performed depending on the size of training sample and correlation properties of clutter. The comparison of simulation and calculation results for indirect and direct algorithms revealed their complete match and confirmed the asymptotic efficiency of resultant estimates and the equivalence of indirect and direct algorithms.References
SKOLNIK, M.I. (ed.), Radar Handbook, 3rd ed. McGraw-Hill, 2008.
RICHARDS, M.A.; SCHEER, J.A.; HOLM, W.A. (eds.), Principles of Modern Radar: Basic Principles. New York: SciTech Pub., IET, Edison, 2010.
POPOV, D.I. “An adaptation of nonrecursive rejectors,” Radioelectron. Commun. Syst., v.52, n.4, p.197, 2009. DOI: https://doi.org/10.3103/S0735272709040050.
LOZOVSKYI, I.F. “Construction and efficiency of adaptive signal processing in conditions of multiple noises,” Uspekhi Sovremennoi Radioelektroniki. Zarubezhnaya Radioelektronika, n.1, p.52, 2016. URI: http://www.radiotec.ru/article/7230#english.
MELVIN, W.L.; SCHEER, J.A. (eds.), Principles of Modern Radar: Advanced Techniques. New York: SciTech Publishing, IET, Edison, 2013.
RICHARDS, M.A. Fundamentals of Radar Signal Processing, 2nd ed. New York: McGraw-Hill Education, 2014.
POPOV, D.I.; SMOLSKIY, S.M. “Estimation of the clutter correlation coefficient in radar systems,” Infocommun. J., v.VIII, n.3, p.8, Sept. 2016.
PEROZZI, G.; EFIMOV, D.; BIANNIC, J.-M.; PLANCKAERT, L.; COTON, P. “Wind estimation algorithm for quadrotors using detailed aerodynamic coefficients,” Proc. of Annual American Control Conf., ACC, 27-29 Jun. 2018, Milwaukee, USA. IEEE, 2018, p.1921-1926. DOI: https://doi.org/10.23919/ACC.2018.8431879.
MALIKOV, E.; SUN, Y. “Semiparametric estimation and testing of smooth coefficient spatial autoregressive models,” J. Econometrics, v.199, n.1, p.12, 2017. DOI: https://doi.org/10.1016/j.jeconom.2017.02.005.
TARIMA, S. “Statistical estimation in the presence of possibly incorrect model assumptions,” J. Statistical Theory Practice, v.11, n.3, p.449, 2017. DOI: https://doi.org/10.1080/15598608.2017.1299056.
KARMAKAR, B.; MUKHOPADHYAY, I. “Risk efficient estimation of fully dependent random coefficient autoregressive models of general order,” Commun. Statistics - Theory Methods, v.47, n.17, p.4242, 2018. DOI: https://doi.org/10.1080/03610926.2017.1371758.
SUN, Y.; MALIKOV, E. “Estimation and inference in functional-coefficient spatial autoregressive panel data models with fixed effects,” J. Econometrics, v.203, n.2, p.359, 2018. DOI: https://doi.org/10.1016/j.jeconom.2017.12.006.
KOSHEVOI, V.M.; MEDVEDIK, A.D. “Development of estimators of clutter parameters based on circuits of alternate period compensation,” Radioelectron. Commun. Syst., v.23, n.12, p.82, 1980.
OLGUIN, C.J.M.; SAMPAIO, S.C.; DOS REIS, R.R. “Statistical equivalence of prediction models of the soil sorption coefficient obtained using different log P algorithms,” Chemosphere, v.184, p.498, 2017. DOI: https://doi.org/10.1016/j.chemosphere.2017.06.027.
PEPELYSHEV, A.; KORNIKOV, V.; ZHIGLJAVSKY, A. “Statistical estimation in global random search algorithms in case of large dimensions,” in: R. Battiti, D. Kvasov, Y. Sergeyev (eds.), Learning and Intelligent Optimization. LION 2017. Lecture Notes in Computer Science, v.10556. Cham; Springer, 2017. DOI: https://doi.org/10.1007/978-3-319-69404-7_32.
HUA, X.; CHENG, Y.; WANG, H.; QIN, Y. “Information geometry for covariance estimation in heterogeneous clutter with total Bregman divergence,” Entropy, v.20, n.4, p.258, 2018. DOI: https://doi.org/10.3390/e20040258.
LAZRIEVA, N.; TORONJADZE, T. “Recursive estimation procedures for one-dimensional parameter of statistical models associated with semimartingales,” Trans. A. Razmadze Math. Institute, v.171, n.1, p.57, 2017. DOI: https://doi.org/10.1016/j.trmi.2016.12.001.
POPOV, D.I. “Adaptive clutter rejection,” Radioelectron. Commun. Syst., v.56, n.4, p.186, 2013. DOI: https://doi.org/10.3103/S0735272713040031.
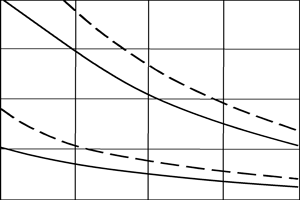