Wavelet filtering by using nonthreshold method and example of model Doppler function
DOI:
https://doi.org/10.3103/S0735272721070049Keywords:
wavelet filtering, Doppler effect, correlation distance, Doppler model function, entropy errorAbstract
The paper is devoted to the comparative analysis of the efficiency of two methods of discrete wavelet filtering. The first method consists in zeroing of the detailing coefficients up to a specific decomposition level, the determination of which was not investigated in the available publications, while the second method is often applied in practice and involves the use of common constrained threshold of detailing coefficients for all the decomposition levels.
For finding the level of wavelet decomposition ensuring the minimal filtering error in time domain, the root-mean-square error of model was employed. In this case, the cosine and correlation distances reflect the filter performance efficiency as was revealed on the basis of results of their comparison with Euclidean norms of vectors in frequency domain.
The analysis of wavelet filtering efficiency implies the need to divide the plane of noise distribution laws into two areas: one with the laws close to the normal distribution and the second with all the other laws. For the first group of noises with distribution close to normal, the relationship of the filtering error as a function of the decomposition level features a pronounced extreme character (i.e., minimum) making it possible to design simple filters with minimal computational costs by using the minimum error criterion. The comparison of the proposed filtering method with the classical Butterworth filter resulted in obtaining the identical errors with other factors being equal.
References
R. Martinek, J. Zídek, “Use of adaptive filtering for noise reduction in communications systems,” in 2010 International Conference on Applied Electronics, 2010, uri: https://ieeexplore.ieee.org/document/5599607.
S. K. Mishra, D. K. Upadhyay, M. Gupta, “Optimized first-order s-to-z mapping function for IIR filter designing,” in 2019 20th International Conference on Intelligent System Application to Power Systems (ISAP), 2019, pp. 1–5, doi: https://doi.org/10.1109/ISAP48318.2019.9065934.
H. Liu, W. Wang, C. Xiang, L. Han, H. Nie, “A de-noising method using the improved wavelet threshold function based on noise variance estimation,” Mech. Syst. Signal Process., vol. 99, pp. 30–46, 2018, doi: https://doi.org/10.1016/j.ymssp.2017.05.034.
Y. Zhang, W. Ding, Z. Pan, J. Qin, “Improved wavelet threshold for image de-noising,” Front. Neurosci., vol. 13, 2019, doi: https://doi.org/10.3389/fnins.2019.00039.
Q. Lu et al., “High-G calibration denoising method for high-G MEMS accelerometer based on EMD and wavelet threshold,” Micromachines, vol. 10, no. 2, p. 134, 2019, doi: https://doi.org/10.3390/mi10020134.
C. A. Langston, S. M. Mousavi, “Separating signal from noise and from other signal using nonlinear thresholding and scale‐time windowing of continuous wavelet transforms,” Bull. Seismol. Soc. Am., vol. 109, no. 5, pp. 1691–1700, 2019, doi: https://doi.org/10.1785/0120190073.
I. M. Johnstone, B. W. Silverman, “Empirical Bayes selection of wavelet thresholds,” Ann. Stat., vol. 33, no. 4, pp. 1700–1752, 2005, doi: https://doi.org/10.1214/009053605000000345.
H. Zhang, T. R. Blackburn, B. T. Phung, D. Sen, “A novel wavelet transform technique for on-line partial discharge measurements. 1. WT de-noising algorithm,” IEEE Trans. Dielectr. Electr. Insul., vol. 14, no. 1, pp. 3–14, 2007, doi: https://doi.org/10.1109/TDEI.2007.302864.
Y. E. Voskoboynikov, D. A. Krysov, “Wavelet-filtration of noise different statistical nature,” Mod. High Technol., no. 6, pp. 50–54, 2018, uri: https://top-technologies.ru/ru/article/view?id=37032.
Y. E. Voskoboynikov, “Wavelet filtering with two parametric threshold functions: selection of the function and justification of optimal parameters,” Avtom. i Program. Inzheneriya, no. 1, pp. 69–78, 2016, uri: http://jurnal.nips.ru/sites/default/files/АИПИ-1-2016-8.pdf.
Q. Huang et al., “Application of a novel constrained wavelet threshold denoising method in ensemble-based background-error variance,” Sci. China Technol. Sci., vol. 61, no. 6, pp. 809–818, 2018, doi: https://doi.org/10.1007/s11431-016-9098-3.
G. Sheng, G. Gao, B. Zhang, “Application of improved wavelet thresholding method and an RBF network in the error compensating of an MEMS gyroscope,” Micromachines, vol. 10, no. 9, p. 608, 2019, doi: https://doi.org/10.3390/mi10090608.
N. Zhang, P. Lin, L. Xu, “Application of weak signal denoising based on improved wavelet threshold,” IOP Conf. Ser. Mater. Sci. Eng., vol. 75112073, 2020, doi: https://doi.org/10.1088/1757-899X/751/1/012073.
Y. Shen, G. Zhao, S. Zhang, S. Li, Y. Li, “Image denoising method and evaluation based on mixed wavelet algorithm,” in Eleventh International Conference on Digital Image Processing (ICDIP 2019), 2019, p. 141, doi: https://doi.org/10.1117/12.2540098.
H.-Y. Gao, A. G. Bruce, “Waveshrink with firm shrinkage,” Stat. Sin., vol. 7, no. 4, pp. 855–874, 1997.
H. Qiu, J. Lee, J. Lin, G. Yu, “Wavelet filter-based weak signature detection method and its application on rolling element bearing prognostics,” J. Sound Vib., vol. 289, no. 4–5, pp. 1066–1090, 2006, doi: https://doi.org/10.1016/j.jsv.2005.03.007.
B. S. Gurevich, S. B. Gurevich, V. V. Manoilov, “Wavelet filtering of spatial frequencies during sampling of light fields,” Nauchnoe Priborostr., vol. 22, no. 1, pp. 101–106, 2012.
G. Lee, R. Gommers, F. Waselewski, K. Wohlfahrt, A. O’Leary, “PyWavelets: a Python package for wavelet analysis,” J. Open Source Softw., vol. 4, no. 36, p. 1237, 2019, doi: https://doi.org/10.21105/joss.01237.
I. M. Korzhov, “Analysis of models of coherence of spectral non-stationality of random signals,” Bull. Natl. Tech. Univ. «KhPI». Ser. Hydraul. Mach. Hydraul. Units, no. 46, pp. 30–34, 2019, uri: http://repository.kpi.kharkov.ua/handle/KhPI-Press/39950.
O. Y. Oliynyk, Y. K. Taranenko, “Analysis of signal coherence using wavelet transform method,” Metrol. Instruments, no. 6, pp. 48–52, 2020.
P. V. Novitskii, “Concept of an error entropy value,” Meas. Tech., vol. 9, no. 7, pp. 872–875, 1966, doi: https://doi.org/10.1007/BF00998440.
O. Oliynyk, Y. Taranenko, D. Losikhin, A. Shvachka, “Examining the Kalman filter in the field of noise and interference with the non-Gaussian distribution,” Eastern-European J. Enterp. Technol., vol. 4, no. 4 (94), pp. 36–42, 2018, doi: https://doi.org/10.15587/1729-4061.2018.140649.
E. V. Burnaev, N. N. Olenev, “Proximity measure for time series on basis of wavelet coefficients,” in Proc. of XLVIII Scientific Conference of MFTI, 2005.
S. Mahata, R. Kar, D. Mandal, “Direct digital fractional-order Butterworth filter design using constrained optimization,” AEU - Int. J. Electron. Commun., vol. 128, p. 153511, 2021, doi: https://doi.org/10.1016/j.aeue.2020.153511.
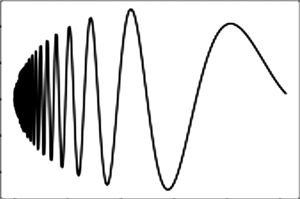