Discrete LS estimates of correlation function of bi-periodically correlated random signals
DOI:
https://doi.org/10.3103/S0735272720030036Keywords:
bi-periodically correlated random process, correlation function estimation, least squares method, asymptotical unbiasedness, asymptotical consistency, leakage effect, discretization stepAbstract
Analysis of discrete estimates of bi-periodically correlated random processes (BPCRP)—mathematical models of signals with double stochastic periodicity was performed using least squares (LS) technique. It is shown that LS utilization allows to avoid systematic errors related to leakage effect. Expressions for estimate bias and dispersion were obtained, allowing determination systematic and mean square errors depending on discretization step, sample number and signal parameters were obtained. For quadrature BPCRP model discrete and continuous dispersions of LS estimation of correlation components were compared. Recommendations for discretization step are given.References
A. M. Yaglom, Correlation Theory of Stationary Random Functions with Examples from Meteorology [in Russian] (Leningrad, Gidrometeoizdat, 1981).
Jacques Max, Jean-Louis Lacoume, Méthodes et Techniques de Traitement du Signal [in French] (Dunod, Malakoff, 2004).
J. S. Bendat, A. G. Piersol, Random Data, Analysis and Measurement Procedures (New York, Wiley-Interscience, 1986).
W. A. Gardner, Ed., Cyclostationarity in Communications and Signal Processing. New York: IEEE Press, 1994.
H. L. Hurd and A. Miamee, Periodically Correlated Random Sequences: Spectral Theory and Practice. New Jersey: Wiley-Interscience, 2007.
W. A. Gardner, A. Napolitano, and L. Paura, “Cyclostationarity: Half a century of research,” Signal Processing, vol. 86, no. 4. pp. 639–697, 01-Apr-2006, doi: https://doi.org/10.1016/j.sigpro.2005.06.016.
A. Napolitano, “Cyclostationarity: New trends and applications,” Signal Process., vol. 120, pp. 385–408, 2016, doi: https://doi.org/10.1016/j.sigpro.2015.09.011.
I.M. Javorskyj, Mathematical Models and Analysis of Stochastic Oscillations [in Ukrainian] (Lviv, PMI NASU, 2013).
J. Antoni, “Cyclostationarity by examples,” Mechanical Systems and Signal Processing, vol. 23, no. 4. Academic Press, pp. 987–1036, 01-May-2009, doi: https://doi.org/10.1016/j.ymssp.2008.10.010.
W. A. Gardner, “Exploitation of Spectral Redundancy in Cyclostationary Signals,” IEEE Signal Process. Mag., vol. 8, no. 2, pp. 14–36, 1991, doi: https://doi.org/10.1109/79.81007.
C. Capdessus, M. Sidahmed, J. L. Lacoume, “Cyclostationary processes: application in gear faults early diagnosis,” Mech. Syst. Signal Process., v.14, n.3, p.371, 2000. DOI: https://doi.org/10.1006/mssp.1999.1260.
I. Javorskyj, I. Kravets, I. Matsko, and R. Yuzefovych, “Periodically correlated random processes: Application in early diagnostics of mechanical systems,” Mech. Syst. Signal Process., vol. 83, pp. 406–438, 2017, doi: https://doi.org/10.1016/j.ymssp.2016.06.022.
A. Napolitano, Generalizations of Cyclostationary Signal Processing: Spectral Analysis and Applications. Wiley-IEEE Press, 2012.
A. Napolitano, Cyclostationary Processes and Time Series: Theory, Applications, and Generalizations. Cambridge, MA: Academic Press, 2019.
I. Javorskyj, I. Kravets, R. Yuzefovych, I. G. Stetsko, I. Matsko, “Development of information-measuring system for multidimensional diagnostics of rotating units of machine complexes,” in: Resource and Safety Operation Issues of Structures, Buildings, and Machines [in Ukrainian] (Kyiv, IEW NASU, 2012).
I. M. Javorskyj, R. M. Yuzefovych, O. Y. Dzeryn, “LSM-harmonic analysis of bi-periodic nonstationary vibration signals,” Information Extraction and Processing, n.45, p.14, 2017. URI: http://vidbir.ipm.lviv.ua/vidbir-article-2017-121-3.htm>.
I. M. Javorskyj, O. Y. Dzeryn, and R. M. Yuzefovych, “Covariance LSM-analysis of biperiodic nonstationary vibration signals,” Inf. Extr. Process., vol. 2018, no. 46, pp. 19–28, 2018, doi: https://doi.org/10.15407/vidbir2018.46.019.
I. Javors’kyj, I. Matsko, R. Yuzefovych, and Z. Zakrzewski, “Discrete estimators of characteristics for periodically correlated time series,” Digit. Signal Process. A Rev. J., vol. 53, pp. 25–40, 2016, doi: https://doi.org/10.1016/j.dsp.2016.03.003.
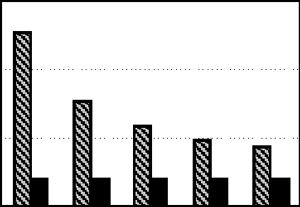