Detection efficiency of signal with unknown non-power parameter using algorithms based on the Compressive Sensing theory
DOI:
https://doi.org/10.3103/S0735272718080046Keywords:
orthogonal matching pursuit, compressive sensing, detection algorithm, likelihood ratio, sufficient statistic, non-power parameter, sparse signal, total probability of error, ideal observer criterion, signal-to-noise ratio, correlation functionAbstract
The problem of detecting quasi-deterministic signals against the background of noise during the digital processing has been considered. In this case, at the specified detection efficiency, the minimum criterion of required arithmetic operations was used as a synthesis criterion for such algorithms. To this end, such algorithms were synthesized based on concepts of the compressive sensing theory. The computer simulation was used to check the efficiency of developed algorithms making it possible to determine the manner in which the total probability of detection errors depends on the signal-to-noise ratio and the compression ratio (ratio of the number of elements in the sufficient statistic vectors before and after “compression”). The detection efficiency losses of proposed algorithms were determined in comparison with the classical optimal algorithm in accordance with the maximum likelihood method at different values of signal-to-noise ratio and compression ratio. At the same time, the paper indicates the gain in the number of used arithmetic operations of proposed algorithms as compared to the classical one. The presented results allow us to make a sound choice of detection algorithm depending on the available hardware resources and admissible degradation of the detection efficiency.References
HELSTROM, C. Statistical Theory of Signal Detection. New York: Pergamon Press, 1960.
VAN TREES, H.L. Detection, Estimation, and Modulation Theory, Part I. New York: Wiley, 1968; Part III. New York: Wiley, 1971.
AKIMOV, P.S.; BAKUT, P.A.; BOGDANOVICH, V.A. Theory of Signal Detection [in Russian, ed. by P. A. Bakuta]. Moscow: Radio i Svyaz’, 1984.
SHAKHTARIN, B.I. Signal Detection: Manual for Graduate Students, 3rd ed. [in Russian]. Moscow: Goryachaya Liniya–Telekom, 2015.
VISHNEVSKII, V.M.; LYAKHOV, A.I.; PORTNOI, S.L.; SHAKHNOVICH, I.V. Wideband Wireless Information Transfer Networks [in Russian]. Moscow: Tekhnosfera, 2005.
CANDES, E.J.; WAKIN, M.B. “An introduction to compressive sampling,” IEEE Signal Processing Mag., v.25, n.2, p.21-30, 2008. DOI: https://doi.org/10.1109/MSP.2007.914731.
DONOHO, D.L. “Compressed sensing,” IEEE Trans. Inf. Theory, v.52, n.4, p.1289-1306, 2006. DOI: https://doi.org/10.1109/TIT.2006.871582.
ELDAR, Y.C.; KUTYNIOK, G. Compressed Sensing: Theory and Applications. Cambridge University Press, 2012.
FOUCART, S.; RAUHUT, H. A Mathematical Introduction to Compressive Sensing. Springer, 2013. DOI: http://doi.org/10.1007/978-0-8176-4948-7.
PARFENOV, V.I.; GOLOVANOV, D.Y. “Detection of vacant unoccupied frequency sub-bands by compressed measurements,” Infokommunikacionnye Tehnologii, v.13, n.3, p.305-312, 2015. DOI: https://doi.org/10.18469/ikt.2015.13.3.11.
PARFENOV, V.I.; GOLOVANOV, D.Y. “Principles of construction and effectiveness analysis of operation of wireless sensor networks based on compressive sensing theory,” Digital Signal Processing, n.2, p.14-19, 2016.
PARFENOV, V.I.; GOLOVANOV, D.Y. “Detection of discrete sparse signals with a sampling rate not exceeding the Nyquist frequency,” J. Radio Electronics, n.6, 2017. URI: https://jre.cplire.ru/jun17/1/abstract.html.
DRAGANIC, A.; OROVIC, I.; STANKOVIC, S. “On some common compressive sensing recovery algorithms and applications,” Facta universitatis - series: Electronics and Energetics, v.30, n.4, p.477-510, 2017. DOI: https://doi.org/10.2298/FUEE1704477D.
ABO-ZAHHAD, M.M.; HUSSEIN, A.I.; MOHAMED, A.M. “Compressive sensing algorithms for signal processing applications: a survey,” Int. J. Commun., Network System Sci., v.8, n.6, p.197-216, 2015. DOI: http://doi.org/10.4236/ijcns.2015.86021.
ALLEN-ZHU, Z.; GELASHVILI, R.; RAZENSHTEYN, I. “Restricted isometry property for general p-norms,” IEEE Trans. Inf. Theory, v.62, n.10, p.5839-5854, 2016. DOI: https://doi.org/10.1109/TIT.2016.2598296.
STRANG, G. Linear Algebra and Its Applications. New York: Academic Press, 1976.
TROPP, J.A.; GILBERT, A.C. “Signal recovery from random measurements via orthogonal matching pursuit,” IEEE Trans. Inf. Theory, v.53, n.12, p.4655-4666, 2007. DOI: https://doi.org/10.1109/TIT.2007.909108.
CAI, T.T.; WANG, L. “Orthogonal matching pursuit for sparse signal recovery with noise,” IEEE Trans. Inf. Theory, v.57, n.7, p.4680-4688, 2011. DOI: https://doi.org/10.1109/TIT.2011.2146090.
WANG, J.; SHIM, B. “Exact recovery of sparse signals using orthogonal matching pursuit: how many iterations do we need?,” IEEE Trans. Signal Processing, v.64, n.16, p.4194-4202, 2016. DOI: https://doi.org/10.1109/TSP.2016.2568162.
NEEDELL, D.; TROPP, J.A. “CoSaMP: Iterative signal recovery from incomplete and inaccurate samples,” Applied and Computational Harmonic Analysis, v.26, n.3, p.301-321, 2009. DOI: https://doi.org/10.1016/j.acha.2008.07.002.
STURM, B.L.; CHRISTENSEN, M.G. “Comparison of orthogonal matching pursuit implementations,” Proc. of 20th European Signal Processing Conf., EUSIPCO, 27-31 Aug. 2012, Bucharest, Romania. IEEE, 2012, p.220-224. URI: https://ieeexplore.ieee.org/document/6333943/.
BERINDE, R.; INDYK, P.; RUZIC, M. “Practical near-optimal sparse recovery in the L1 norm,” Proc. of 46th Annual Allerton Conf. on Communication, Control, and Computing, 23-26 Sept. 2008, Urbana-Champaign, IL, USA. IEEE, 2008, p.198-205. DOI: https://doi.org/10.1109/ALLERTON.2008.4797556.
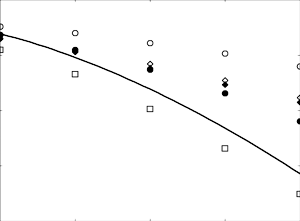